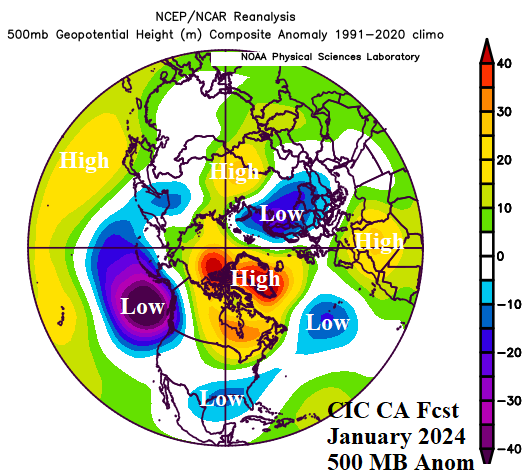
Upper Air Forecast for January 2024 in the Northern Hemisphere
12/22/2023, 1:16 pm EST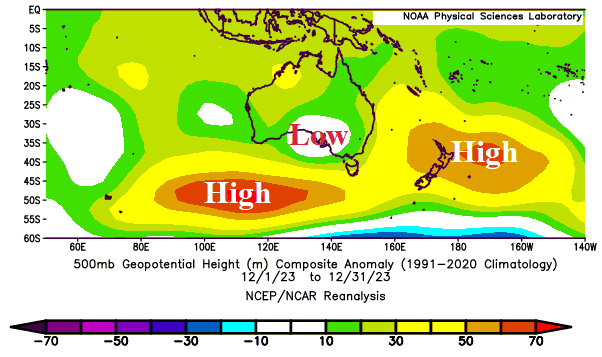
Marine Heatwaves And East Australia Heavy Rains
01/03/2024, 12:44 pm ESTA Short Summary of AI in Weather Prediction and Its Use as a Tool for Forecast Development
Olivia Kellner, Ph.D. Lead Research Scientist
Climate Impact Company
Synopsis: AI-driven weather forecast models trained on data from ERA5 show promise in replicating ECMWF’s Integrated Forecast System (IFS) forecasts in the short-term horizon (0-48 hours) with low Root Mean Square Error (RMSE) values. Computationally significantly less demanding compared to conventional Numerical Weather Prediction models (NWP), AI-driven forecast models still face the same limitation of decay in forecast accuracy the farther the forecast time frame from the initial forecast period.
Introduction: Artificial Intelligence (AI) has entered the world of weather prediction, and there are many questions regarding its strengths and weaknesses compared to the NWP models that have provided hourly, daily, weekly, and monthly forecasts for decades. It is important to note that the approach of AI versus NWP to forecast weather is distinctly different. However, the two approaches share similar fundamental approaches to weather forecasting. Both require an initialization forecast, and both sets of equations (NWP versus AI) that drive the forecast, are fine-tuned using historic weather observations. Additionally, both approaches are iterative. This means that they complete the same forecast process for each step of the forecast using generated forecast output as input for the next iterative step in the forecast process. The use of this approach results in accumulating forecast errors and a decrease in forecast accuracy as the forecast advances beyond the initial state. Where the two forecast approaches differ is the use of the fundamental equations of the atmosphere (NWP) versus the algorithms of AI. This difference is what adds value to AI-driven forecasts. AI-driven forecasts are not nearly as computationally demanding as the partial differential equations that drive NWP. This provides a distinct advantage to AI-based forecasts, especially those models that generate accurate forecasts in the short-term (0-48 hours) when high-impact systems may be unfolding across a given forecast area.
Artificial Intelligence: AI is the scientific field that combines rich, detailed datasets and the world of computer science and its machines to enable systematic problem-solving by a computer. AI includes the sub-fields of Machine Learning (hereafter, ML, which requires a labeled dataset provided by humans to train the algorithm (Supervised Learning)) and Deep Learning (hereafter, DL, which can apply Supervised Learning or classify data on its own (Unsupervised Learning)).
ML and DL are two different approaches that seek to organize data to explain the “why” behind the data existence, or make a prediction based on the input data provided to train the algorithm (i.e., the algorithm is the governing equation or mathematical equations/functions developed to solve the problem/make the prediction).
Lastly, there are Neural Networks (NN) or Artificial Neural Networks (ANN), that mimic the neural pathways of the human brain via node layers with conditionals or thresholds. If the input data to that node meets the conditional/threshold value, it allows for pathway progression/activation to the next node, or if there is no connection established, that node layer progression stops and seeks an alternative node route. DL is a subfield of NN.
“Deep” is used to describe the number of node layers driving a NN, with three or more layers qualifying a NN as a “Deep” Learning network. Less than three layers often referred to as a “Classic” NN and requires more human intervention to train the model.
AI Models available via ECMWF: The European Center for Medium Range Weather Forecasts (ECMWF) shares AI-generated forecasts from four different AI models whose aim is to forecast short- to medium-range weather forecasts. The four models are: FuXi, GraphCast, Pangu-Weather, and FourCastNet. All four AI-based models are developed or “trained” using the ECMWF Reanalysis Version 5.0 (ERA5) dataset (1940 – present, hourly + temporal resolution).
The reader is referred to the following papers for the details of each model, with the following table highlighting model specifics. All models provide forecasts of atmospheric variables such as wind speeds, temperatures, atmospheric pressure fields, surface variables, relative humidity, and/or vertically integrated total column of water vapor, and/or precipitation like conventional NWP models:
- Pathak, J. et al., 2022: FourCastNet: A global data-driven high-resolution weather model using adaptive Fourier neural Operators;
- Bi, K., et al., 2022: Pangu-Weather: A 3D High-resolution system for fast and accurate global weather forecast;
- Lam, R., et al., 2023: GraphCast: Learning skillful medium-range global weather forecasting; and
- Chen, L., et al., 2023: FuXi: A cascade machine learning forecasting system for 15-day global weather forecast.
Model | Spatial Resolution | Temporal Resolution | Focused Forecast Time Frame |
Pangu-Weather | 0.25° x 0.25° | Hourly | 0 – 7 days |
FuXi | 0.25° x 0.25° | 6-hourly | 0 – 5, 5 – 10, 10 – 15 days |
FourCastNet | 0.25° x 0.25° | 6-hourly | 0 – 7 days |
GraphCast | 0.25° x 0.25° | 6-hourly | 0 – 10 days |
ECMWF has published a blog article that compares the four models to the IFS, IFS Ensemble mean, and ECMWF’s own AI-driven model, the Artificial Intelligence/Integrated Forecasting System (AIFS). The article can be found here: https://www.ecmwf.int/en/about/media-centre/aifs-blog/2023/new-ml-model-ecmwf-web-charts. The figure below is from that article. In looking at the RMSE of all four models, as well as AIFS against the IFS and ensemble mean, for a forecast timeframe of June 1, 2023, to August 31, 2023, of 850 hPa temperatures for the Northern Hemisphere, one can see that AI-based models perform as well as conventional NWP (i.e., the IFS). But as time progresses in the forecast, the RMSE increases for 850 hPa temperatures (you want a low RMSE). This means, that just like NWP, the forecast accuracy of AI-driven models diminishes as time progresses from the initial forecast state.
From the article: “A new ML model in the ECMWF web charts” by Zied Ben Bouallegue and the AIFS team published on 13 December 2023. The above image shows “Difference with respect to IFS statistics: a) RMSE (the lower, the better) and (b) forecast activity (the higher, the more active) for temperature at 850 hPa (T850) averaged over 1 June 2023 to 31 August 2023, northern hemisphere.”
It is important to note that the four AI-drive models (FuXi, GraphCast, FourCastNet, and Pangu-weather) all take a distinctly different approach to model development. Pangu-weather creates a 3-D space within its forecast model and applies a “hierarchal temporal aggregation to alleviate forecast errors.” One can see the improvement in the Pangu-Weather model accuracy via the blue line in the image above, which maintains the lower RMSE value through forecast lead times. FuXi is also a strong performer but has a distinct decline in RMSE values once it progresses from the fine-tuned 0-5 day forecast model state to the next fine-tune forecast state of 5-10 days. This is not to say that FourCastNet and GraphCast are not strong performers. It is to highlight that the unique structure of each model will provide strengths and weaknesses compared to each other, just like all NWP models.
Concluding Remarks: AI-driven weather forecast models show strong achievement in reducing the amount of time required to create a weather forecast, as the models are not driven by the computationally demanding fundamental equations of the atmosphere like NWP. However, like NWP, weather forecasts generated by AI-driven models struggle with forecast accuracy at longer lead times, due to the iterative approach of weather forecasting to atmospheric variables that are chaotic and stochastic. AI does not surpass NWP in forecast accuracy and remains in its primary years in application to weather prediction. It should be used as a forecast tool in conjunction with NWP, not a stand-alone resource for weather forecasts. The coming years will include AI-driven forecast model improvements and refinements, as well as expansion into forecasts of extreme weather events exacerbated by anthropogenic climate change due to AI’s unique ability to “train” on weather observation data of recent decades. Weather observations which demonstrate increased intensity from a warming climate system.
References:
- “A new ML model in the ECMWF web charts”: https://www.ecmwf.int/en/about/media-centre/aifs-blog/2023/new-ml-model-ecmwf-web-charts
- FuXi: https://arxiv.org/pdf/2306.12873.pdf
- GraphCast: https://arxiv.org/pdf/2212.12794.pdf
- Pangu-Weather: https://arxiv.org/pdf/2211.02556.pdf
- FourCastNet: https://arxiv.org/pdf/2202.11214.pdf